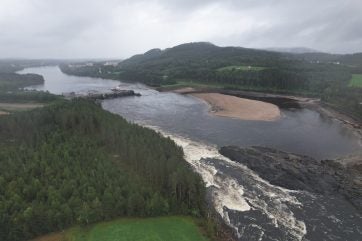
There was a time in the not-too-distant past when insurance companies refused to pay for what they called “acts of God”, which in turn included almost all natural catastrophes, and which were considered rare enough to be unpredictable and beyond the ability of humans to prevent. Nowadays, based on probabilistic risk analysis methods, we know that no natural hazard turns into a disaster, much less a catastrophe, unless supplemented by human factors, specifically, by the infrastructural and societal vulnerabilities, as well as by the exposure of assets and human beings.
Furthermore, weather extremes and hydrological hazards, which seem to be intensifying over the years, are at least partially human-caused, via climate and land use change. The latter situation has been occasionally providing an excuse for inaction: lack of investments in critical infrastructure and human development, as well as inadequate economic investments and financial instruments, are being brushed under the carpet by blaming all weather and hydrological disasters as “acts of climate change”. Other than effectively replacing “God” with “climate change”, it has become all too convenient for local governments and national agencies, which are usually charged with, and happen to be in the best position to invest in, disaster risk reduction and climate adaptation, to blame climate-related disasters on the lack of mitigation and financing at international scales.
The truth, meanwhile, is a bit more nuanced. In the interests of this article, let us discuss if we can search for the elusive truth by examining three broad cases, specifically, dam construction, dam operations, and hydropower management.
Dam constructors and operators, as well as hydropower and river managers, fulfil critical societal responsibilities by saving lives and reducing property damage from flood and drought disasters, and by ensuring the supply of clean water and energy to homes and businesses. However, they are being increasingly challenged by relatively rare or even unprecedented extremes and stresses, as well as by the superposition of fluctuations and natural variability with long-term trends.
Grey swans and butterflies
Nicholas Nasser Taleb, a Wall Street trader, introduced the concept of swans in the assessments of risks and resilience. White swan events are not-too-unexpected minor shocks that do not disrupt human or societal functions. Black swan events are surprising and rare to the point of being unpredictable and out of bounds for probabilistic descriptions – although on hindsight they may appear inevitable – with deep and lasting impacts.
Grey swans are predictive surprises: these events may be relatively rare, but they can be characterised probabilistically, and while they may be unprecedented in a region, the combination of process changes and data-driven understanding make them viable in the future. Thus, climate change may cause grey swan storms and cyclones, while a combination of climate change with lack of investments in techno-social readiness and high exposure in vulnerable regions may lead to grey swan flooding disasters.
Ed Lorenz, an MIT meteorologist, introduced the concept of chaos or butterfly effects to weather prediction. The extreme sensitivity to initial conditions (with relatively abrupt decay in predictability beyond certain lead times) and the presence of what are called strange attractors in dynamic plots of weather variables (which in certain idealised situations look like butterfly wings), was paraphrased for non-specialists by Lorenz: “When a butterfly flutters its wings in one part of the world, it can eventually cause a hurricane in another.”
This butterfly effect makes weather extremes and hydrologic disasters difficult to predict (and hence to prepare for with precision) beyond certain lead times. This is true even with the best of prediction tools, although advances in process models and data-driven methods can enable the forecaster to better approach the theoretical bounds to predictability. While butterfly effects were supposed to be less dominant at climate scales (to the point that climate model simulations did not even run too many initial condition simulations previously), we now know that natural variability (or extreme sensitivity to initial conditions) is a characteristic of global climate and earth system models as well. This realization has exacerbated the ensemble multiplier effect in earth system models, where in addition to emissions pathways and multiple models, ensembles need to be generated for initialisations as well.
Furthermore, we are beginning to characterise the extent to which climate change may increase variability in weather or hydrologic stresses and extremes. While variability and predictability are not identical concepts, increasing variability in climate and weather may lead to lack of predictability in projections.
A combination of grey swans (or the probabilistic viability of relatively rare or even unprecedented extremes) and butterfly effects (or the theoretical bounds to predictability and need for initial-condition ensembles) have serious implications for dam and hydropower constructors, owners, managers, operators, and stakeholders. The construction of dams and hydraulic infrastructures needs to consider both the changes in the intensity (severity) or depth and duration and frequency (IDF or DDF) curves of precipitation and flooding (or drought) extremes, as well as the natural variability and predictability of hydrometeorological systems and extremes through the design life of infrastructures.
Operations and management of dams need to account for the drought-and-deluge-cycles, especially the possibility of unprecedented (yet viable owing to changes in the underlying causal drivers) extremes, and the loss of predictability manifested in different ways from lead times of a few hours to a few weeks, as well as from interannual to decadal scales. Hydropower managers need to be ready to handle changes in extremes, whether heavy precipitation and flash droughts, and longer-term changes in the statistics of precipitation including heavy rainfall and drought events, as well as changes in the patterns of water-related stresses, whether caused by changes in the demand and supply of water or by changes in water temperature.
The amplification of threats and risks caused by grey swans and the limits to predictability imposed by butterfly effects needs to be addressed by a suite of tools and strategies.
First, there is a need to develop better tools for catastrophe modeling which accounts for grey swans and butterfly effects based on physics-grounded extreme value statistics, nonlinear dynamical principles for large model ensembles, and effective blending of process understanding with data-driven methods including artificial intelligence and machine learning.
Second, performance-based engineering, which includes design and operations, must be developed to make infrastructures resilient, in a way that optimises risk-informed engineering with cost or frugality constraints. Besides explicit controls for costs, flexible planning, operations, and design, including grey-green solutions can help, especially for longer-term adaptation to changing design and operations curves.
Third, advances in risk modeling and resilient design needs to be developed in tandem with economic or financial instruments, as well as governance and policy tools and strategies, for holistic management and preparedness.
Interested readers may want to look at [1-12] for further reading.
Acknowledgment
Funding was provided by NASA ROSES and Water Resources programmes for the Category 1 project “Remote-sensing data driven Artificial Intelligence for precipitation-Nowcasting (RAIN).” The author thanks the project contributors and partners include Puja Das and August Posch from NU, Nathan Barber, and Michael Hicks from the Tennessee Valley Authority (TVA), Thomas Vandal and Kate Duffy from the NASA SBIR funded startup Zeus AI (both NU PhD alums), Debjani Singh of the US DOE’s Oak Ridge National Laboratory (ORNL), and Katie van Werhoven of the RTI. The climate downscaling and variability work benefited from the work by former SDS Lab PhD students Kate Duffy, Thomas Vandal, Devashish Kumar, Udit Bhatia and Evan Kodra, among others, while the hydropower and dam construction insights benefited from prior work by PhD alumni Udit Bhatia, Nishant Yadav, and Evan Kodra, and by postdoctoral alumna Poulomi Ganguli.
References
[1] Horsburgh, K., Haigh, I.D., Williams, J., De Dominicis, M., Wolf, J., Inayatillah, A. and Byrne, D., 2021. “Grey swan” storm surges pose a greater coastal flood hazard than climate change. Ocean Dynamics, 71(6-7), pp.715-730. [2] Lin, N. and Emanuel, K., 2016. Grey swan tropical cyclones. Nature Climate Change, 6(1), pp.106-111. [3] Zhang, Y., Long, M., Chen, K., Xing, L., Jin, R., Jordan, M.I. and Wang, J., 2023. Skilful nowcasting of extreme precipitation with NowcastNet. Nature, 619(7970), pp.526-532. [4] Deser, C., Lehner, F., Rodgers, K.B., Ault, T., Delworth, T.L., DiNezio, P.N., Fiore, A., Frankignoul, C., Fyfe, J.C., Horton, D.E. and Kay, J.E., 2020. Insights from Earth system model initial-condition large ensembles and future prospects. Nature Climate Change, 10(4), pp.277-286. [5] Kumar, D. and Ganguly, A.R., 2018. Intercomparison of model response and internal variability across climate model ensembles. Climate dynamics, 51(1-2), pp.207-219. [6] Krishnamurti, T.N., Kishtawal, C.M., LaRow, T.E., Bachiochi, D.R., Zhang, Z., Williford, C.E., Gadgil, S. and Surendran, S., 1999. Improved weather and seasonal climate forecasts from multimodel superensemble. Science, 285(5433), pp.1548-1550. [7] Vandal, T., Kodra, E., Ganguly, S., Michaelis, A., Nemani, R. and Ganguly, A.R., 2017, August. Deepsd: Generating high resolution climate change projections through single image super-resolution. In Proceedings of the 23rd acm sigkdd international conference on knowledge discovery and data mining (pp. 1663-1672). [8] Liu, Y., Ganguly, A.R. and Dy, J., 2020, August. Climate downscaling using YNet: A deep convolutional network with skip connections and fusion. In Proceedings of the 26th ACM SIGKDD International Conference on Knowledge Discovery & Data Mining (pp. 3145-3153). [9] Aerts, J.C., Botzen, W.W., Emanuel, K., Lin, N., De Moel, H. and Michel-Kerjan, E.O., 2014. Evaluating flood resilience strategies for coastal megacities. Science, 344(6183), pp.473-475. [10] Ganguly, A.R., Bhatia, U. and Flynn, S.E., 2018. Critical infrastructures resilience: Policy and engineering principles. Routledge. 154 pages. [11] Northeastern Global News (September 12, 2023, by Tanner Stening): Flooding in Libya a ‘gray swan’ event, but dam infrastructure worldwide ‘not ready’ to meet the demands of a changing climate, expert says. Available from: https://news.northeastern.edu/2023/09/12/libya-flooding/. [12] Northeastern Global News (December 4, 2023, by Cynthia Hibbert): The short-term rain forecast system is broken. Can AI do a better job of predicting deadly floods? Available from: https://news.northeastern.edu/2023/12/04/flood-prediction-artificial-intelligence/.About the author
Auroop Ratan Ganguly, a college of engineering distinguished professor at Northeastern University (NU) in Boston, MA, with a joint appointment as a chief scientist at the US Department of Energy’s Pacific Northwest National Laboratory in Richland, WA, has over 25 years of full-time professional experience in the US spanning the private industry, government research laboratory, and academia. Ganguly has published widely in water and climate science and engineering, critical infrastructure resilience, as well as machine learning, nonlinear dynamics, and extreme value statistics.
He has co-founded or advised two successful startups in climate and weather analytics, led or supported community science related to climate action in the greater Boston area, and has been collaborating with scientists in US, Europe, India, Indonesia, Brazil, Tanzania, and around the world.
At NU, Ganguly directs the Sustainability and Data Sciences Laboratory within Civil and Environmental Engineering, the Artificial Intelligence for Climate and Sustainability focus area within the Institute for Experiential AI and until recently, was one of two Co-Directors leading the Global Resilience Institute. He is a Fellow of the American Society of Civil Engineers, a Distinguished Member of the Association for Computing Machinery and obtained a PhD in hydrology from the CEE Department at the Massachusetts Institute of Technology (MIT) in Cambridge, MA.
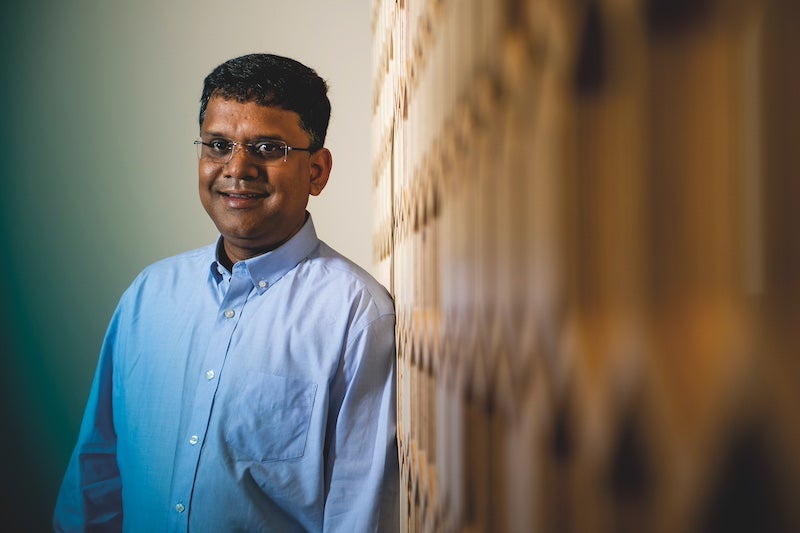