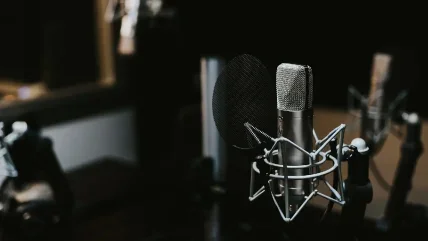
Please introduce us to Upstream Tech
At Upstream Tech, our mission is to build software products that make cutting-edge remote sensing and machine learning technology accessible and easy to use, in order to accelerate the pace, scale, and impact of environmental work around the globe. Upstream Tech was founded by Marshall Moutenot and Alden Keefe Sampson, two computer science engineers with a vision to use data for the greater good and create accessible pathways for organisations to advance how they use technology for effective decision making.
What is HydroForecast?
Upstream Tech’s HydroForecast is a water forecasting product that provides organisations with actionable weather and water data for operations and planning. HydroForecast’s accuracy in streamflow forecasting is due to how the underlying model blends physical hydrology, principles and theories, with the power of machine learning and data science. This “theory-guided machine learning” hybrid approach enables HydroForecast to leverage the latest advancements in remote sensing data and hydrologic research into a seamless cloud-based operational system for organisations to use. Built on global datasets, HydroForecast can be deployed anywhere, and we are currently operational with customers on five continents.
HydroForecast has been described as taking a novel approach to streamflow forecasting – why is this?
Traditional hydrologic models require a significant amount of upfront work to collect data, calibrate and evaluate before deployment so each time you go to a new place, you have to set up a new model. And if there are changes in the basin, eg a forest fire, or restoration, these models need recalibration.
Our underlying technology is a theory-guided machine learning model. What this means is that we integrate tools from both physical and statistical methodologies to build HydroForecast. We put together what we know about physical hydrology from academic research and from folks on our team who have experience in hydrology and understand the principles and theories, and then we use a neural network model to put all the data together and make our forecasts. This hybrid approach enables us to take advantage of the latest advancements in computer resources, remote sensing data, and hydrologic research– something unique in our field.
As we deal with more frequent extremes, with certain parts of the world seeing weather and streamflow events they have never seen, our model is a bridge that connects these methodologies together and is free of the constraints of needing detailed field measurements (physical) or needing to rely on historical data relationships (statistical).
What are the advantages of using HydroForecast?
HydroForecast is flexible and well-suited to tackling questions around climate resiliency. The model takes advantage of recent developments in machine learning that are particularly well-suited for making sense of the complicated relationships between the variables that affect flows, like precipitation and temperature. As historic relationships between weather (eg snow) and runoff are changing, this model is capable of dynamically adapting to learn these changes.
HydroForecast receives information from multiple weather forecast sources, including the National Oceanic and Atmospheric Administration in Washington, D.C and its European equivalent’s leading predictions. The model is trained on multiple weather forecasts so that when it goes into real-time operations, it has seen and learned the biases and errors in the forecasts and can use that knowledge to make a better overall prediction. Ultimately, this gives users a more thorough picture of what might happen in the coming days and the uncertainty ahead, like whether or not a big storm will hit a specific place.
Satellite imagery and incorporation of any available streamflow measurements show the model’s near real-time information about the current conditions on the ground in a watershed. This means that its predictions are regularly ‘ground-truthed’ to live conditions and allow it to adapt automatically to changes in the basin that affect how water flows, including conditions like forest fires and urbanisation.
Can you give examples in the hydropower and water industry where Hydroforecast has been used to date and what it has achieved?
In a live forecasting competition co-hosted by the US Bureau of Reclamation and the CEATI Hydropower Interest Group over water year 2021, across 19 sites in North America and 25 categories of performance metrics, HydroForecast took 23 of the 25 first place wins and was the strongest overall forecast in each region.
For hydropower users, HydroForecast can increase annual revenue between 3-6%, according to a study by the Idaho National Laboratory. Upstream Tech shared its forecasts with the research team to test if improved reservoir inflow forecasts could enable operators to meet additional constraints while maintaining or increasing revenue. The study found that during the highest revenue months, using HydroForecast increased asset revenue by 6% over operations informed by a persistence forecast. In addition, the study found that better forecasts can enable operators to improve environmental outcomes at their asset without sacrificing revenue.
We’ve also shown that HydroForecast’s seasonal (90-day) water supply forecast outperformed the California Department of Water Resources over the past two spring periods at the five largest reservoirs that control much of California’s water supply and allocation. In a specific instance at the North Fork American River at North Fork Dam in Calionria, HydroForecast accurately predicted a very difficult rain on snow event. Forecasts were accurately updated multiple times per day to track dynamically changing hydrologic conditions which aided smooth operations as the flows peaked and receded. With HydroForecast, water managers were able to de-risk decisions leading up to the difficult-to-forecast rain on snow event.
Any other comments?
Building on the strengths of our core model, we have developed the capability to predict hydrologic scenarios out to 2100 through HydroForecast Long-term.
HydroForecast Long-term is our solution to the complex challenge of arming those making decade-scale decisions with the best possible information about the future of water, temperature, and precipitation. It makes it easy to break the cycle of relying on past conditions to indicate what the future will look like. Most existing process-based hydrologic models are not well suited to adapt to these new complexities and basin changes since they were built on assumptions between inputs and output that no longer hold true as the climate warms. HydroForecast Long-term leverages the same theory-guided machine learning approach found in our operational forecasts with the addition of temperature and precipitation projections from global climate models. The result is a probabilistic view of water supply over the next decades that gives organisations confidence to make climate-resilient decisions. We look forward to sharing more about HydroForecast Long-term soon.